Genomics: Insight
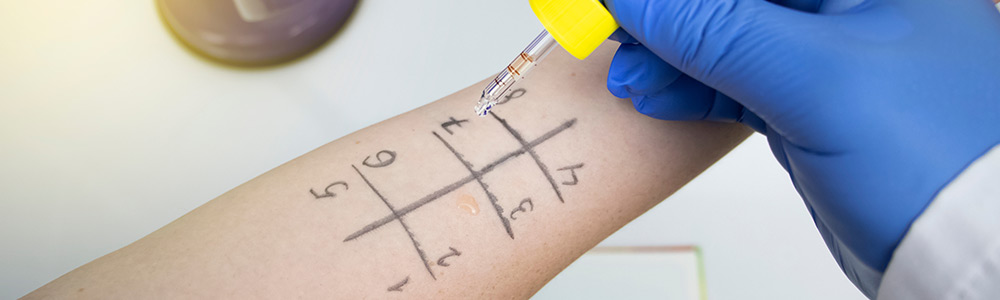
Machine Learning and Food Allergy
Advancing Diagnosis and Quality of Care
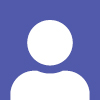
Abstract:
How can machine learning improve food allergy diagnosis and quality of life? Food allergies represent a significant global health concern, with increasing prevalence and severe impacts on quality of life. Potentially dangerous oral-food challenges, inconsistent skin-prick tests, and the lack of consumer-friendly allergen assays highlight a need for innovation in allergy therapeutics, which can be improved through machine learning (ML). This review highlights genetic predisposition and the selection of aptamers, which are short DNA or RNA molecules that selectively bind specific targets, like allergenic proteins. There are associations drawn between specific genetic loci and food allergies, as well as associations between epigenetic patterns or environmental influences with food allergies. ML algorithms, particularly those based on random forest and neural networks, have shown remarkable accuracy in predicting aptamer-protein interactions. Using the burgeoning field of machine learning in combination with genomics has the potential to dramatically change allergy-related therapeutics.
Mechanisms and Outcomes of Food Allergy:
Food allergy is described as a consistent negative immune reaction to a certain food. [1] The most common type of food allergy is IgE-mediated (immunoglobulin E). Mixed IgE and non-IgE food allergies are characterized by the immune system reacting to certain foods through both IgE-mediated and non-IgE-mediated pathways. Some forms of this allergy include atopic dermatitis or delayed food reactions [2][3][4]. Anaphylaxis, the most severe version of an allergic reaction, has the potential to be fatal. This process can severely compromise the body's physiological functions, leading to possible airway obstruction, cardiovascular collapse, and other organ shock. Food is the most common source of anaphylactic allergic reactions (peanuts, tree nuts, shellfish/fish, cow’s milk, wheat, and eggs). [5] [6] [7]
Food is the most common source of anaphylactic allergic reactions
Global Prevalence of Food Allergy:
Country/Region | Prevalence/Statistic | Year(s) |
---|---|---|
U.S. | 32 million with food allergies | - |
1.6 million children with peanut allergies | - | |
200,000 hospitalizations yearly | - | |
Allergies increased by 50% | Since 1997 | |
Tree nut and peanut allergies tripled | Since 1997 | |
UK | 4% reported food allergy | - |
Hospital admissions from anaphylaxis: 1.2 to 2.4 per 100,000 | 1998 to 2005 | |
Denmark | 3.6% reported food allergy | - |
Norway | 6.8% reported food allergy | - |
Australia | >10% at age 12 months | - |
Anaphylaxis admission annual increase: 13.2% | 1994 to 2005 | |
Thailand | 1% - 3.8% for children 3 to 7 years | - |
China | 7.7% of 1- and 2-year-old children | - |
Anaphylaxis admission increase: 3.5% to 7.7% | 1999 to 2009 | |
Ghana | 11% reported food allergy | - |
Japan, Taiwan, Hong Kong, South Korea | 3.4% to 7.0% self-reported allergies | - |
Africa (various) | 5% - 19% self-reported allergies | - |
Effects of Food Allergy on Quality of Life:
Children with allergies experience negative impacts on their quality of life, such as being harassed because of their food allergy, intentionally isolating themselves, or exhibiting signs of PTSD from anaphylaxis. [13] [14] [15] [16] [17]. 24% of all food-allergic teens and adults have been harassed at some point because of their food allergy. [18] The cost of the EpiPen, which delivers life-saving medication during anaphylactic shock, is also rising in price, faster than the rate of CPI inflation. [19] The costs of treating allergy-related incidents and hospitalizations have a $24.8 billion economic impact. [20] Families with children from the lowest income groups have to spend more than twice as much as those in higher income groups for food allergy emergencies and hospitalization costs. [21] There is no current consumer-friendly test for preventing accidental consumption of food allergens.
There is no current consumer-friendly test for preventing accidental consumption of food allergens.
Limitations of Current Food Allergy Diagnostics:
The current forms of diagnosing IgE food allergy are skin-prick tests, where a patient is pricked with a small amount of allergen-containing liquid. The sensitivity is determined by the extent of skin swelling, known as the wheal. If the wheal is under a certain diameter, an oral food challenge is recommended, as the allergy is likely mild. [22] Skin tests, though, have a low specification for clinically significant food allergy. As a result, people are characterized through a skin-prick test as having a mild allergy, even though they may have a dangerous allergy. The oral food challenge that follows is dangerous and fatal, leading to possible gastrointestinal, cutaneous oral, upper respiratory, and lower respiratory reactions, as well as anaphylaxis [23] [24].
Genetic and Epigenetic Markers Associated with Food Allergy:
In a genome-wide association study, Hong et al. discovered many loci associated with food allergy. Two loci associated highly with peanut allergy were found near genes HLA-DQB1, HLA-DQA2, and HLA-DRA, which are associated with the human leukocyte antigen (HLA) complex. This complex plays a critical role in the immune system. The prevalence of these genes was frequent since the minor allele frequencies (MAFs), or frequency at which the less common allele occurred, ranged from 0.31 to 0.39 (Max is 0.5). For egg allergy, two single-nucleotide-polymorphisms were found close to genes SGCD (involved in maintaining integrity of muscles) and ITIH5 (involved in stability of the extracellular matrix and inflammatory response), with MAFs around 0.07 and 0.39, respectively. Milk allergy was associated with two SNPs near the genes FAM117A (unclear function) and LSP1 (involved in white-blood cell function) (MAFs of 0.15 and 0.03). The differing MAF values in the same type of food allergy suggest that genetic variation is still individualized, making a single-gene association for all food-allergy unlikely. [25]
MAF values in the same type of food allergy suggest that genetic variation is still individualized
Martino et al. analyzed epigenetic factors associated with food allergy, specifically on how gene expression and methylation differed in activated versus quiescent (inactive) CD4+ T cells for children with IgE-mediated food allergies. CD4+ T cells are a type of white blood cell that sends signals to other types of immune cells. They found many gene sets involved in cell cycle regulation, metabolism, and immune response that were expressed more in active CD4+ T cells. E2F and MYC transcription factor networks showed increased activity, with 213 and 215 genes upregulated, respectively. (P value of 0.00, FDR of 0.00). The gene sets identified in this study could be used for diagnosing food allergy. However, the sample size of this study focused on infants, reducing generalizability. [26]
One study used machine learning to determine associations between methylation patterns and food allergy, to be used as possible epigenetic biomarkers [27]. The analysis narrowed down 405,000+ potential CpG biomarkers to 18, which aligned to genes like Ring Finger Protein 213 (role in tagging proteins for degradation) and Seryl-TRNA Synthetase (enzyme that attaches Serine to tRNA) (frequency-26 times). This study proved that modern machine learning techniques could be used in conjunction with epigenetics to identify biomarkers of food allergy. However, the samples used to identify these biomarkers were only studied in people known to have food allergy, and not compared to those without it.
Impact of Machine Learning on Allergen Diagnosis: Improved Aptamer-Based Allergen Detection
Machine learning has a big possible impact on aptamer selection, for improving diagnostic tests for allergies. Aptamers are a strand of nucleotides that can form a specific binding affinity for molecules based on their secondary structure. To find a specific aptamer, one must go through SELEX, which is an arduous process of binding, rinsing, and amplification between potential aptamers and a specific target. Machine learning can help sort through and select, or even get rid of the need for large libraries of possible aptamers to use during SELEX. A computer program that can predict binding would prove invaluable for creating protein-aptamer-diagnostic tests. Many food allergens are proteins found in foods.
Random forest-based machine learning was used to predict the interaction between aptamers and proteins. Importantly, this research associated genetic material with the conventional and the pseudo amino acid composition of a target protein. [28] Bashir et al. used a particle display to separate a library of aptamers by affinity and used that data to train machine learning models to predict affinity, predicting aptamer candidates at a rate 11-fold higher than random perturbation [29] Tobia et al. developed a new algorithm that combined conserved primary sequences and secondary structures to predict aptamers. [30] Emami et al. developed a new neural network known as AptaNet, which predicted aptamer–protein interaction pairs accounting for many physical factors of the aptamers and proteins. All of the studies advanced the field of aptamer candidate selection by combining machine learning and bioinformatics. The improvement in aptamer-protein interaction prediction can be used for allergen-based assays, where a patient can test their food for unknown contaminants and allergies.
Predicting aptamer candidates at a rate 11-fold higher than random perturbation
Future Steps for Machine Learning:
A model can be trained using the pre-existing genetic and epigenetic biomarkers. This new model should combine age and ancestry as variables, and the biases can be proportional to the prevalence of the genetic biomarker in existing data. However, the new combination of fields of machine learning and genomics, as Sen et al. point out, does not come without consequences. There need to be methods for reducing the inherent biases of training datasets and keeping a wide spectrum of data while balancing the transparency and privacy of participants' data [31] Lastly, the diversity of the sample size for many biomarker-related studies can influence the generalizability of the study, which is important to note when creating this new, all-encompassing model.
Connections to Author and Society:
A way to genetically test and quantify my peanut allergy would be invaluable.
I suffer from bad allergies. I have not done a peanut oral food challenge due to differing results from multiple skin-prick tests; anaphylaxis could happen. I have visited the hospital multiple times for anaphylaxis. A way to genetically test and quantify my peanut allergy would be invaluable. Moreover, a way to test my food before I eat it would also prove beneficial because eating out causes the stress of possible anaphylactic shock (cross-contamination or faulty communication.) Using the burgeoning field of machine learning and computer algorithms in combination with genomics has the potential to dramatically change allergy-related therapeutics and the quality of life for someone with an allergy.
References:
- Moore, L. E., Stewart, P. H., & deShazo, R. D. (2017). Food allergy: What we know now. The American Journal of the Medical Sciences, 353(4), 353–366. https://doi.org/10.1016/j.amjms.2016.11.014
- Moore, L. E., Stewart, P. H., & deShazo, R. D. (2017). Food allergy: What we know now. The American Journal of the Medical Sciences, 353(4), 353–366. https://doi.org/10.1016/j.amjms.2016.11.014
- Bardana, E. J. (2004). Immunoglobulin E‐ (IGE) and non‐ige‐mediated reactions in the pathogenesis of atopic eczema/dermatitis syndrome (AEDS). Allergy, 59(s78), 25–29. https://doi.org/10.1111/j.1398-9995.2004.00565.x
- Rosenstreich, D. L. (1993). Evaluation of delayed hypersensitivity: From PPD to Poison ivy. Allergy and Asthma Proceedings, 14(6), 395–400. https://doi.org/10.2500/108854193778792830
- Fischer, D., Vander Leek, T. K., Ellis, A. K., & Kim, H. (2018). Anaphylaxis. Allergy, Asthma & Clinical Immunology, 14(S2). https://doi.org/10.1186/s13223-018-0283-4
- Nuñez-Borque, E., Fernandez-Bravo, S., Yuste-Montalvo, A., & Esteban, V. (2022). Pathophysiological, cellular, and molecular events of the vascular system in anaphylaxis. Frontiers in Immunology, 13. https://doi.org/10.3389/fimmu.2022.836222
- Kemp, S. F., & Lockey, R. F. (2002). Anaphylaxis: A review of Causes and Mechanisms. Journal of Allergy and Clinical Immunology, 110(3), 341–348. https://doi.org/10.1067/mai.2002.126811
- Gupta, R. S., Warren, C. M., Smith, B. M., Blumenstock, J. A., Jiang, J., Davis, M. M., & Nadeau, K. C. (2018). The public health impact of parent-reported childhood food allergies in the United States. Pediatrics, 142(6). doi:10.1542/peds.2018-1235
- Gupta, R. S., Warren, C. M., Smith, B. M., Jiang, J., Blumenstock, J. A., Davis, M. M., … Nadeau, K. C. (2019). Prevalence and severity of food allergies among us adults. JAMA Network Open, 2(1). doi:10.1001/jamanetworkopen.2018.5630
- Clark, S., Espinola, J., Rudders, S. A., Banerji, A., & Camargo, C. A. (2011). Frequency of US emergency department visits for food-related acute allergic reactions. Journal of Allergy and Clinical Immunology, 127(3), 682–683. doi:10.1016/j.jaci.2010.10.040
- LJ;, J. K. L. (2013). Retrieved from https://pubmed.ncbi.nlm.nih.gov/23742874/
- Loh, W., & Tang, M. (2018). The epidemiology of food allergy in the global context. International Journal of Environmental Research and Public Health, 15(9), 2043. https://doi.org/10.3390/ijerph15092043
- Walkner, M., Warren, C., & Gupta, R. S. (2015). Quality of life in food allergy patients and their families. Pediatric Clinics of North America, 62(6), 1453–1461. doi:10.1016/j.pcl.2015.07.003
- Feng, C., & Kim, J.-H. (2018). Beyond avoidance: The psychosocial impact of Food Allergies. Clinical Reviews in Allergy & Immunology, 57(1), 74–82. doi:10.1007/s12016-018-8708-x
- Weiss, D., & Marsac, M. L. (2016). Coping and posttraumatic stress symptoms in children with food allergies. Annals of Allergy, Asthma & Immunology, 117(5), 561–562. doi:10.1016/j.anai.2016.08.022
- Ersig, A. L., Brown, R. L., & Malecki, K. (2021). Association between food allergy, Psychological stress, and Allostatic Load. Western Journal of Nursing Research, 44(10), 946–954. doi:10.1177/01939459211019042
- King, R. M., Knibb, R. C., & Hourihane, J. O. (2009). Impact of peanut allergy on quality of life, stress and anxiety in the family. Allergy, 64(3), 461–468.
- Lieberman, J. A., Weiss, C., Furlong, T. J., Sicherer, M., & Sicherer, S. H. (2010). Bullying among pediatric patients with food allergy. Annals of Allergy, Asthma & Immunology, 105(4), 282–286. doi:10.1016/j.anai.2010.07.011
- Westermann-Clark, E., Pepper, A. N., & Lockey, R. F. (2022). Anaphylaxis. Immunology and Allergy Clinics of North America, 42(1), 175–186. doi:10.1016/j.iac.2021.09.004
- Gupta, R., Holdford, D., Bilaver, L., Dyer, A., Holl, J. L., & Meltzer, D. (2013b). The economic impact of childhood food allergy in the United States. JAMA Pediatrics, 167(11), 1026. doi:10.1001/jamapediatrics.2013.2376
- Warren, C. M., Jiang, J., & Gupta, R. S. (2020b). Epidemiology and burden of food allergy. Current Allergy and Asthma Reports, 20(2). doi:10.1007/s11882-020-0898-7
- Peters, R. L., Gurrin, L. C., & Allen, K. J. (2011). The predictive value of Skin Prick testing for challenge‐proven food allergy: A systematic review. Pediatric Allergy and Immunology, 23(4), 347–352. https://doi.org/10.1111/j.1399-3038.2011.01237.x
- Perry, T. T., Matsui, E. C., Conover-Walker, M. K., & Wood, R. A. (2004). Risk of oral food challenges. Journal of Allergy and Clinical Immunology, 114(5), 1164–1168. https://doi.org/10.1016/j.jaci.2004.07.063
- Cianferoni, A., Khullar, K., Saltzman, R., Fiedler, J., Garrett, J. P., Naimi, D. R., & Spergel, J. M. (2013). Oral Food Challenge to wheat: A near-fatal anaphylaxis and review of 93 food challenges in children. World Allergy Organization Journal, 6, 14. https://doi.org/10.1186/1939-4551-6-14
- Hong, X., Hao, K., Ladd-Acosta, C., Hansen, K. D., Tsai, H.-J., Liu, X., Xu, X., Thornton, T. A., Caruso, D., Keet, C. A., Sun, Y., Wang, G., Luo, W., Kumar, R., Fuleihan, R., Singh, A. M., Kim, J. S., Story, R. E., Gupta, R. S., … Wang, X. (2015). Genome-wide association study identifies peanut allergy-specific loci and evidence of epigenetic mediation in US children. Nature Communications, 6(1). https://doi.org/10.1038/ncomms7304
- Martino, D., Neeland, M., Dang, T., Cobb, J., Ellis, J., Barnett, A., Tang, M., Vuillermin, P., Allen, K., & Saffery, R. (2018). Epigenetic dysregulation of naive CD4+ T-cell activation genes in childhood food allergy. Nature Communications, 9(1). https://doi.org/10.1038/s41467-018-05608-4
- Alag, A. (2019). Machine learning approach yields epigenetic biomarkers of food allergy: A novel 13-gene signature to diagnose clinical reactivity. PLOS ONE, 14(6). https://doi.org/10.1371/journal.pone.0218253
- Manju, N., Samiha, C. M., Kumar, S. P., Gururaj, H. L., & Flammini, F. (2022). Prediction of aptamer protein interaction using random forest algorithm. IEEE Access, 10, 49677–49687. https://doi.org/10.1109/access.2022.3172278
- Bashir, A., Yang, Q., Wang, J., Hoyer, S., Chou, W., McLean, C., Davis, G., Gong, Q., Armstrong, Z., Jang, J., Kang, H., Pawlosky, A., Scott, A., Dahl, G. E., Berndl, M., Dimon, M., & Ferguson, B. S. (2021). Machine learning guided aptamer refinement and Discovery. Nature Communications, 12(1). https://doi.org/10.1038/s41467-021-22555-9
- Perez Tobia, J., Huang, P.-J. J., Ding, Y., Saran Narayan, R., Narayan, A., & Liu, J. (2023). Machine learning directed aptamer search from conserved primary sequences and secondary structures. ACS Synthetic Biology, 12(1), 186–195. https://doi.org/10.1021/acssynbio.2c00462
- Sen, S. K., Green, E. D., Hutter, C. M., Craven, M., Ideker, T., & Di Francesco, V. (2024). Opportunities for basic, clinical, and bioethics research at the intersection of Machine Learning and Genomics. Cell Genomics, 4(1), 100466. https://doi.org/10.1016/j.xgen.2023.100466
About the Author
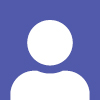
Theodore Kratter is a student at Polytechnic. He is currently researching allergy-related diagnostic assays. His research interests include allergies, therapeutics, nutrition, synthetic biology, and subatomic physics. He enjoys baking various breads and playing water polo.